Congress:
ECR25
Poster Number:
C-14730
Type:
Poster: EPOS Radiologist (scientific)
Authorblock:
G. Skouroumouni1, K. Loizidou2, G. Savvidou2, A. Constantinidou2, E. Orphanidou Vlachou2, A. Yiallourou2, C. Pitris2, C. T. Nikolaou1; 1Limassol/CY, 2Nicosia/CY
Disclosures:
Galateia Skouroumouni:
Nothing to disclose
Kosmia Loizidou:
Nothing to disclose
Gabriella Savvidou:
Nothing to disclose
Anastasia Constantinidou:
Nothing to disclose
Eleni Orphanidou Vlachou:
Nothing to disclose
Anneza Yiallourou:
Nothing to disclose
Costas Pitris:
Nothing to disclose
Christos Th Nikolaou:
Nothing to disclose
Keywords:
Breast, Mammography, Computer Applications-General, Cancer
The clinical application of this algorithm has the potential to significantly improve the management of breast cancer, with increased vigilance, ultimately leading to earlier detection, timely intervention, and improved patient outcomes.
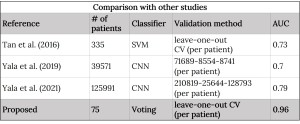
Table 3: Comparison of other algorithms for the prediction of breast cancer using sequential mammograms.
Limitations
- Data collection is very challenging since three consecutive rounds of mammograms are needed for each participant along with biopsy confirmations and detailed annotations.
- Relatively small dataset with unbalanced data.
- Potential differences in annotations, when more experts evaluate the same mammograms.
Future Work
- Larger dataset that will include a broader age range and more representative samples.
- Since most hospitals are now embracing digital breast tomosynthesis (DBT) along with mammography for patients with suspicious findings, the proposed technique should be extended to DBT.
- Deep learning should be evaluated using both mammograms and DBT.
Acknowledgments
The publication of this paper is supported by the European Union’s Horizon 2020 research and innovation programme under grant agreement No 739551 (KIOS CoE) and the Government of the Republic of Cyprus through the Cyprus Deputy Ministry of Research, Innovation and Digital Policy.
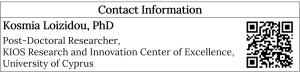