Patient Demographics and Radiograph Characteristics
In this study, radiograph sets from 119 patients were analyzed, with a median age of 38 years (interquartile range [Q1-Q3]: 26-56.5 years; range: 16-89 years). Of these patients, 52 (43.7%) were female. The radiographs were evaluated for the presence of abnormalities, with 29 (24.4%) of the sets identified as abnormal. Among these abnormal sets, 10 (37.9%) were classified as critical in nature, and 17 (58.6%) contained a non-nodular pulmonary opacity.
Overall Performance of the AI Algorithm
The AI algorithm succeeded in interpreting all the 119 radiograph sets of the study dataset. It demonstrated a high level of accuracy in its analysis of the chest radiographs. 3 abnormal radiograph sets were missed, and 3 normal sets were incorrectly identified as abnormal, yielding an overall accuracy of 95%, a sensitivity of 88.5%, a specificity of 96.8%, a positive predictive value of 88.5%, and a negative predictive value of 96.8%. Examples of AI results are provided in Figure 1, 2, 3 and 4.
When focusing specifically on critical findings, the pooled accuracy, Se, Sp, PPV and NPV were of 96.6%, 80%, 98.2%, 80%, and 98.2% respectively. For relevant findings, the pooled performances were slightly lower but still robust, with an accuracy of 93.3%, a sensitivity of 84.2%, a specificity of 95%, a PPV of 76.2%, and an NPV of 96.9%.
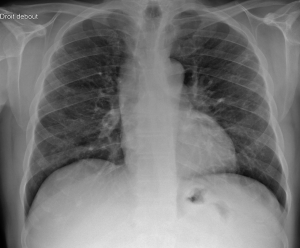
Performance Per Specific Findings
The AI algorithm's performance was particularly noteworthy in its evaluation of pneumothorax and fractures as it did not miss any cases of pneumothorax (n=2) or fractures (n=2), and there were no false positives reported for these findings, reaching a diagnosis performance of 100%. In addition, none of the 2 pulmonary nodule radiograph sets were missed.
Excluding pneumothorax and fractures, the accuracy ranged from 91.6% for non-nodular pulmonary opacity to 96.6% for pulmonary nodules, the NPV ranged from 96.9% for non-nodular pulmonary opacity to 100% for pulmonary nodules.