The AI application demonstrated progressive improvement through the development stages. Initial validation on original MIP images (without ROI definition) achieved an accuracy of 0.91, sensitivity of 0.94, and specificity of 0.88. After implementing ROI definition, the validation performance improved significantly, reaching an accuracy of 0.97, sensitivity of 0.99, and specificity of 0.94. The final evaluation on the independent test set of 40 cases showed exceptional performance, with an accuracy of 0.99, sensitivity of 1.0, and specificity of 0.99, with a rapid processing time of 40 seconds per scan.
To understand the algorithm's decision-making process, GRAD-CAM analysis was performed to visualize the significant pixels contributing to classification decisions. Figure 1 demonstrates a normal case before (a) and after (b) ROI definition, with corresponding GRAD-CAM maps (c,d). Figure 2 shows a ductopenic case with similar visualization arrangement. In the normal case (Figure 1), the algorithm successfully focused on salivary ducts both in original and ROI-defined images. However, in the ductopenic case (Figure 2), the original MIP image analysis showed attention to irrelevant areas including dental restorations such as crowns and roots, while the ROI-defined analysis demonstrated more precise focus on the main salivary duct and its central branching patterns. These findings highlight the importance of proper ROI definition in achieving accurate classification.
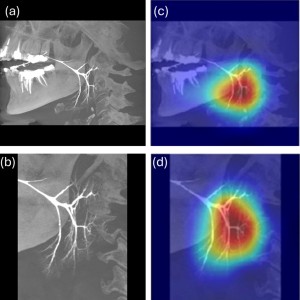
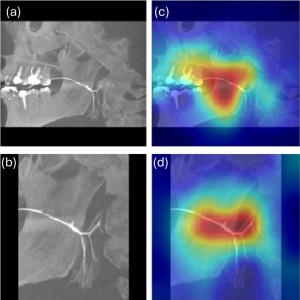