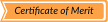
[1] M. Price, C. Ballard, J. Benedetti, C. Neff, G. Cioffi, K.A. Waite, C. Kruchko, J.S. Barnholtz-Sloan, Q.T. Ostrom, CBTRUS Statistical Report: Primary Brain and Other Central Nervous System Tumors Diagnosed in the United States in 2017-2021., Neuro-Oncol. 26 (2024) vi1–vi85. https://doi.org/10.1093/neuonc/noae145.
[2] P. Kickingereder, F. Isensee, I. Tursunova, J. Petersen, U. Neuberger, D. Bonekamp, G. Brugnara, M. Schell, T. Kessler, M. Foltyn, Automated quantitative tumour response assessment of MRI in neuro-oncology with artificial neural networks: a multicentre, retrospective study, Lancet Oncol. 20 (2019) 728–740.
[3] P. Vollmuth, M. Foltyn, R.Y. Huang, N. Galldiks, J. Petersen, F. Isensee, M.J. van den Bent, F. Barkhof, J.E. Park, Y.W. Park, Artificial intelligence (AI)-based decision support improves reproducibility of tumor response assessment in neuro-oncology: an international multi-reader study, Neuro-Oncol. 25 (2023) 533–543.
[4] J.D. Rudie, A.M. Rauschecker, R.N. Bryan, C. Davatzikos, S. Mohan, Emerging applications of artificial intelligence in neuro-oncology, Radiology 290 (2019) 607–618.
[5] K.V. Hoebel, C.P. Bridge, S. Ahmed, O. Akintola, C. Chung, R.Y. Huang, J.M. Johnson, A. Kim, K.I. Ly, K. Chang, Expert-centered Evaluation of Deep Learning Algorithms for Brain Tumor Segmentation, Radiol. Artif. Intell. 6 (2023) e220231.
[6] U. Baid, S. Ghodasara, S. Mohan, M. Bilello, E. Calabrese, E. Colak, K. Farahani, J. Kalpathy-Cramer, F.C. Kitamura, S. Pati, The rsna-asnr-miccai brats 2021 benchmark on brain tumor segmentation and radiogenomic classification, ArXiv Prepr. ArXiv210702314 (2021).
[7] R.W. Cox, J. Ashburner, H. Breman, K. Fissell, C. Haselgrove, C.J. Holmes, J.L. Lancaster, D.E. Rex, S.M. Smith, J.B. Woodward, A (sort of) new image data format standard: NIfTI-1, in: 2004: p. 01.
[8] F. Isensee, M. Schell, I. Pflueger, G. Brugnara, D. Bonekamp, U. Neuberger, A. Wick, H. Schlemmer, S. Heiland, W. Wick, Automated brain extraction of multisequence MRI using artificial neural networks, Hum. Brain Mapp. 40 (2019) 4952–4964.
[9] S. Pati, A. Singh, S. Rathore, A. Gastounioti, M. Bergman, P. Ngo, S.M. Ha, D. Bounias, J. Minock, G. Murphy, The cancer imaging phenomics toolkit (CaPTk): technical overview, in: Springer, 2020: pp. 380–394.
[10] B.K.K. Fields, E. Calabrese, J. Mongan, S. Cha, C.P. Hess, L.P. Sugrue, S.M. Chang, T.L. Luks, J.E. Villanueva-Meyer, A.M. Rauschecker, J.D. Rudie, The University of California San Francisco Adult Longitudinal Post-Treatment Diffuse Glioma MRI Dataset., Radiol. Artif. Intell. 6 (2024) e230182. https://doi.org/10.1148/ryai.230182.
[11] L. Gagnon, D. Gupta, G. Mastorakos, N. White, V. Goodwill, C.R. McDonald, T. Beaumont, C. Conlin, T.M. Seibert, U. Nguyen, J. Hattangadi-Gluth, S. Kesari, J.D. Schulte, D. Piccioni, K.M. Schmainda, N. Farid, A.M. Dale, J.D. Rudie, Deep Learning Segmentation of Infiltrative and Enhancing Cellular Tumor at Pre- and Posttreatment Multishell Diffusion MRI of Glioblastoma, Radiol. Artif. Intell. 6 (2024) e230489.
[12] J. Zhang, D. LaBella, D. Zhang, J.L. Houk, J.D. Rudie, H. Zou, P. Warman, M.A. Mazurowski, E. Calabrese, Development and Evaluation of Automated Artificial Intelligence-Based Brain Tumor Response Assessment in Patients with Glioblastoma, Am. J. Neuroradiol. (2024) ajnr.A8580. https://doi.org/10.3174/ajnr.A8580.
[13] F. Isensee, P.F. Jaeger, S.A.A. Kohl, J. Petersen, K.H. Maier-Hein, nnU-Net: a self-configuring method for deep learning-based biomedical image segmentation, Nat. Methods 18 (2021) 203–211. https://doi.org/10.1038/s41592-020-01008-z.
[14] A. Myronenko, M. Mahfuzur Rahman Siddiquee, D. Yang, Y. He, D. Xu, Automated head and neck tumor segmentation from 3D PET/CT, ArXiv E-Prints (2022) arXiv:2209.10809. https://doi.org/10.48550/arXiv.2209.10809.
[15] T. Rohlfing, D.B. Russakoff, C.R. Maurer, Performance-based classifier combination in atlas-based image segmentation using expectation-maximization parameter estimation, IEEE Trans. Med. Imaging 23 (2004) 983–994.