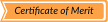
Diffuse gliomas are the most common malignant primary brain tumors in adults and one of the deadliest types of cancer [1]. There are many challenges in treatment and monitoring due to genetic diversity and high intrinsic heterogeneity in appearance, shape, histology, and treatment response. Treatments include surgery, radiation, and systemic therapies, with magnetic resonance imaging (MRI) playing a key role in treatment planning and post-treatment longitudinal assessment. Post-treatment imaging of diffuse gliomas is therefore a fundamental part of patient management that significantly influences clinical decision making and outcomes. Automated segmentation of post-treatment diffuse glioma has significant potential to increase workflow efficiency with more rapid and accurate volumetric assessments for treatment planning [2–4]. Most prior research on brain tumor segmentation has been performed in the pre-treatment setting, with a recent survey finding that 98.3% of published glioma segmentation studies had been performed on preoperative imaging [5]. The 2024 brain tumor segmentation challenge on post-treatment glioma (BraTS-PTG) aims to provide a community standard and benchmark for state-of-the-art automated segmentation models based on the largest expert-annotated post-treatment glioma MRI dataset. Models developed during this challenge will advance the field of automated MRI segmentation and contribute to their integration into clinical practice, ultimately enhancing patient care.