Concerns regarding increasing exposure, of both individual patients, as well as the population as a whole, has lead to one of the principal drivers of improvements of CT image quality: a continuous effort to increase CT image quality, while maintaining dose.
Improving resolution, by detecting with smaller pixels, or reconstructing smaller voxels, of CT acquisition and CT images, will require an increase of dose, to mitigate the otherwise inherent increase of Poisson noise, as a result of the decreased number of photons per voxel (see, e.g. [2])
Super-resolution image reconstruction is the intentional reconstruction of images at higher sampling frequency than acquisition creating an image that appears to have been acquired at higher resolution than is actually the case. Its purpose is to improve the quality of the images by suppressing sampling artefacts. A novel deep-learning implementation (Precise Image Quality Engine, or PIQE) has been trained on a dataset of patient images acquired at 0.25 mm slide width and detector pixel size, in order to improve images acquired at 0.5 mm slice width and pixel size, and has recently been released for clinical . For more information on this particular reconstruction algorithm, see e.g. [1] and [3].
The results presented here have been acquired on a 320-slice Canon Aquilion ONE Insight system. Even though this system also has improved detector performance compared to its predecessors, we believe that overall trends with regard to performance of PIQE may be generalizable to earlier Canon Aquilion ONE Genesis systems, and possibly super-resolution deep-learning reconstruction (SR-DLR) algorithms in general.
In a first step we have scanned a Rando phantom with three cardiac protocols to determine CTDI dose levels (resulting from AEC settings), after which we have used fixed mA to scan Catphan 600 for resolution (modulation transfer function, or MTF) and noise power spectrum (3D NPS) analysis at identical dose levels. We have reconstructed the images with AiCE Cardiac L1, L2, L3 (Mild, Standard and Strong) and PIQE Cardiac L1, L2, L3. Note that the PIQE images have been reconstructed with 1024 × 1024 matrix, at 0.22 mm pixel size, half the pixel size as for AiCE (0.43 mm). Slice thickness was 0.5 mm for both AiCE and PIQE. The scan and reconstruction settings are listed in figure 1.
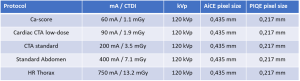
The Catphan images were analysed with pylinac[4] to assess MTF using the line-pair inserts, in addition to Hounsfield Unit (HU) accuracy. The uniform section of the Catphan phantom was used to measure noise, using both standard deviation (s) of an area of 200 × 200 (for AiCE) or 400 × 400 pixels (for PIQE), as well as 3D Noise Power Spectra, using the method of Friedman et al [5], modified for use with CatPhan.