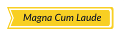
Congress:
ECR24
Poster Number:
C-16391
Type:
EPOS Radiologist (educational)
DOI:
https://dx.doi.org/10.26044/ecr2024/C-16391
Authorblock:
A. Fulga, A. C. Ciurescu, M. Calinescu, B. Popa; Bucharest/RO
Disclosures:
MD Alexandru Fulga:
Nothing to disclose
MD Alexandra Catalina Ciurescu:
Nothing to disclose
Mrs. Miruna Calinescu:
Nothing to disclose
Professor Bogdan Popa:
Nothing to disclose
Keywords:
Artificial Intelligence, Neuroradiology brain, MR, PET-CT, Computer Applications-Detection, diagnosis, Dementia
Read more
Read more
Read more
Read more
Read more