Artificial Intelligence (AI) is increasingly valuable in supporting radiologists, particularly in medical image interpretation, improving diagnostic accuracy, and expediting therapeutic interventions [1]. However, its widespread adoption is still limited by the lack of large annotated datasets, which are challenging to create due to privacy concerns, high costs, and ethical requirements [2, 3]. In light of these challenges, the expansion of datasets with synthetic labeled data is of increasing interest to researchers and clinicians.Generative Adversarial Networks (GANs) [5], offer a promising solution by generating realistic and entirely new medical images that replicate the variability and complexity of the images used in clinical practice. GANs consist of two neural networks, a Generator and a Discriminator, working in a minimax game to improve performance iteratively.
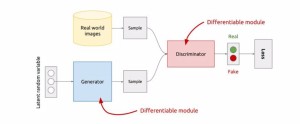
Sun et al. [7] developed HA-GAN, an end-to-end hierarchical architecture generating 256³ resolution images. HA-GAN uses a low-resolution GAN to capture global structure and a high-resolution GAN to refine patches.
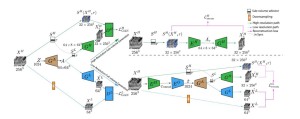
This study addresses the limitations of existing 3D GAN models by proposing CRF-GAN, a novel memory-efficient architecture that combines Conditional Random Fields (CRFs) with GANs to reduce memory usage and improve performance.
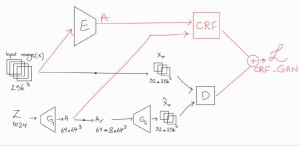
The key contributions of this work are:
- Evaluating CRF-GAN’s clinical value compared to HA-GAN through:(a) Qualitative assessment (primary endpoint).(b) Quantitative assessment (secondary endpoint).
- Developing of a memory-efficient GAN architecture using CRFs to enhance anatomical structure consistency in synthetic images.