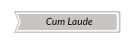
The AI board has successfully overseen the evaluation of all imaging related AI initiatives providing a unified and standardised approach to AI implementation. This is vital in overcoming commonly experienced barriers with the implementation of AI, such as streamlining complex, time consuming information governance approval, upgrading highly variable IT infrastructure, increasing awareness of the transformative potential of AI, and securing funding through industry collaboration10.
During the prioritisation workshop, the AI board identified several key domains for applying AI, such as staff education, request vetting, quality assurance, image interpretation, and patient communication (Figure 3).
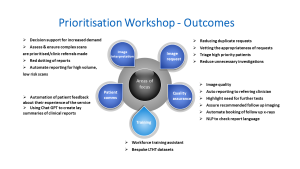
The linked PPIE group consists of 10 members with a mix of gender, age, diversity, and health conditions who play a multifaceted role in assisting the board. The PPIE group have offered unique perspectives on the influence of AI on the patient experience as well as prompting conversations on issues such as ownership, value, and the sharing of patient data with commercial entities. They have provided assistance in communicating with patients regarding the use of AI technology to improve patient acceptability and contributed to the process of prioritisation of AI products for testing and potential deployment.
The setup of an XNAT virtual PACS ‘sandbox’ environment with an AI deployment engine has played a crucial role in enabling efficient retrospective and prospective benchmarking of AI tools. These evaluations are necessary for assessing the efficacy of AI in improving workflow efficiency and diagnostic accuracy prior to clinical deployment as there can be a gap between legal certification and local clinical performance11,12.
The partnerships formed with industry collaborators have been instrumental in leveraging artificial intelligence to enhance radiological operations and patient care. A collaboration with Lucida is being explored as part of a Topol Fellowship feasibility project to assess the role of AI in triaging prostate cancer patients. We have been awarded seed funding from the Yorkshire Imaging Collaborative to evaluate 3 Radiology AI products (Aidoc, Qure.AI and Radiobiotics). In addition, funding for 2 industry-academic collaborations with Mirada Medical and Oxford Heartbeat has been secured from Innovate UK. Collaborations such as these have resulted in academic output supported by industry resources. An important result of this cooperation is the creation of artificial intelligence technologies that are specifically tailored for real world radiological applications. For example, employing AI-assisted PET-CT interpretation in lymphoma treatment response assessment, which has demonstrated potential in reducing interpretation time without compromising accuracy13,14.
A limitation of this project has been that while there is strong clinical support, allocation of IT resources can limit progress. The AI board has allowed clinicians to collaborate and produce efficient solutions to streamline allocation of limited resources and navigate this issue.