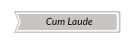
To successfully navigate the complexity associated with the incorporation of AI in clinical radiology while maintaining patient safety, LTHT has established a multidisciplinary clinical AI board. The primary function of this board is to coordinate, supervise, assess all practical implementations of AI solutions and technologies related to imaging and ensure a comprehensive strategy to integrating AI. The board consists of clinical radiologists, radiographers, AI experts from academic and medical image analysis fields, health economists, IT and information governance personnel, and trust management. The AI board collaborates closely with a newly formed patient and public involvement and experience (PPIE) AI group, ensuring that patient viewpoints are incorporated and considered in relation to clinical deployment of AI. A structured framework for AI governance is recommended to deploy and maintain AI models in clinical practice safely and effectively2. The AI board conforms to a comprehensive structured framework with key work packages including information governance/data management; technical rigour, safety and performance; economic and commercial considerations; ethical, medico-legal and privacy consideration. Alongside regular board meetings, a half-day in-person workshop was held in July 2023 to identify priority clinical use cases for imaging AI within the organisation. Participants consisted of members of the AI Board with contributions from clinicians, information technology specialists, radiography professionals, the Association of British HealthTech Industries (ABHI) and patient representatives.
An essential component of our institutional approach to implementation of AI in Radiology involved modifying and employing a scoring matrix derived from a previously published scoring tool, similar to previously described health technology assessments and in line with the AI board key work packages3,4. While there is clear guidance from the Royal College of Radiologists in the UK about how artificial intelligence should integrate with the radiology reporting workflow, at this time there is no guidance to assist institutions with decision making on which AI product to implement5. Our scoring matrix fulfils this unmet need and involves weighted scoring with guidance for key domains such as clinical impact, scientific evidence, workflow impact, ease of use, risk of harm, local performance, maintenance, and monitoring (Figure 1).
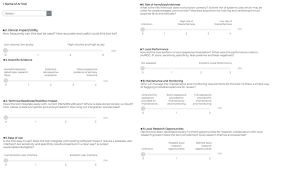
Simultaneously, the growth of our technical infrastructure has been crucial as datasets are required to train, validate, and evaluate AI algorithms. This involved establishment of a secure virtual PACS server utilising the open-source platform Extensible Neuroimaging Archive Toolkit (XNAT). This server, when combined with local clinical expertise facilitates the extraction of rich, representative datasets from our institutional PACS (Figure 2).
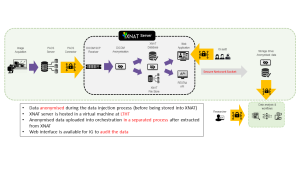
Active collaboration with industry partners has been crucial in aligning AI development with specific clinical requirements, thus expediting the integration of AI technology. We are establishing new collaborations and partnerships to exploit our imaging assets. Support has been provided to companies requiring datasets to develop imaging-based AI diagnostics.