Congress:
ECR24
Poster Number:
C-17087
Type:
EPOS Radiologist (scientific)
DOI:
10.26044/ecr2024/C-17087
Authorblock:
F. Souschek, P. Mildenberger, L. Müller, T. Jorg, M. C. Halfmann; Mainz/DE
Disclosures:
Fabio Souschek:
Nothing to disclose
Peter Mildenberger:
Nothing to disclose
Lukas Müller:
Nothing to disclose
Tobias Jorg:
Nothing to disclose
Moritz Christian Halfmann:
Nothing to disclose
Keywords:
Artificial Intelligence, Thorax, Plain radiographic studies, Comparative studies, Quality assurance
Despite generally lower diagnostic performance in supine chest radiographs compared to PA radiographs, sensitivity and specificity were moderate to high in both groups for detecting lung opacities (85% vs. 92%; 63% vs. 95%), consolidation (80% vs. 75%; 81% vs. 97%), and pleural effusion (77% vs. 87%; 82% vs. 96%, respectively). Lower diagnostic accuracy was observed in detecting pulmonary edema (59% vs. 25%; 61% vs. 100%), pneumothorax (0 % vs. 20%; 100% vs. 100%), and cardiomegaly (21% vs. 46%; 75% vs. 100%).
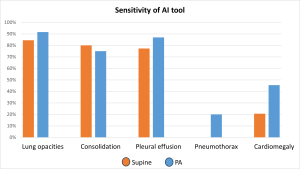
Fig 2: Sensitivity of AI tool
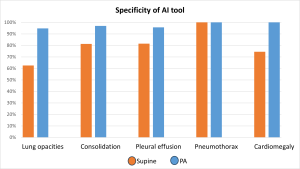
Fig 3: Specificity of AI tool
Positive predictive values were moderate to high for PA radiographs (60-100%) and markedly lower for supine radiographs (0-88%), even though the prevalence of positive target findings was considerably lower for PA radiographs.
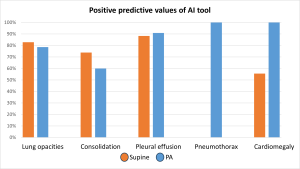
Fig 4: PPV of AI tool
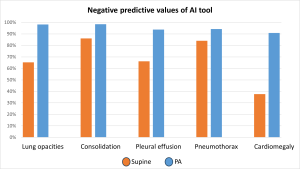
Fig 5: NPV of AI tool