The initial dataset retrieved from the Eurorad library consisted of 4,827 case reports. Using the Llama-3-70B model, we identified and excluded 2,894 cases where the diagnosis was explicitly stated within the case description. The dataset was primarily composed of cases from neuroradiology (21.4%), abdominal imaging (18.1%), and musculoskeletal imaging (14.6%), whereas breast imaging (3.4%) and interventional radiology (1.4%) were underrepresented.
Llama-3-70B exhibited a high accuracy of 87.8% in classifying LLM responses as "correct" or "incorrect" (LLM judge), compared to human expert assessment (123 out of 140 responses; 95% CI: 0.82 – 0.93).
In the Eurorad dataset, GPT-4o demonstrated superior diagnostic performance across all subspecialties except interventional radiology, achieving a rate of 79.6 ± 2.3% correct responses. Meta-Llama-3-70B revealed the highest performance among open-source LLMs (73.2 ± 2.5%), with a considerable margin ahead of Mistral-Small (63.3 ± 2.6%), Qwen2.5-32B (62.5 ± 2.6%), and OpenBioLLM-Llama3-70B (62.5 ± 2.6%). Across all models, the highest levels of diagnostic accuracy were achieved in interventional radiology (67.8 ± 6.2%), cardiovascular imaging (62.5 ± 3.2%), and abdominal imaging (60.5 ± 1.8%).
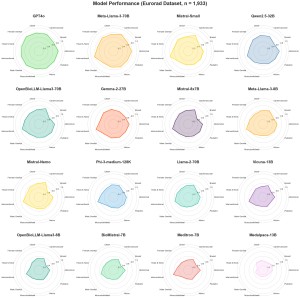
In the local brain MRI dataset, similar results were observed, with GPT-4o (76.7 ± 15.1%) and Llama-3-70B (71.7 ± 12.2%) again leading the rankings. Reader 2, a board-certified neuroradiologist, achieved the highest accuracy with 83.3 ± 13.3% correct responses. Reader 1, a radiologist with 2 years of neuroradiology experience achieved rates comparable to GPT-4o and Meta-Llama-3-70B (75.0 ± 15.5%). Several other models showed a drop in performance levels in the local dataset of up to 16% (e.g. Llama-2-70B: 47.8 ± 2.7% to 31.7 ± 12.6%).
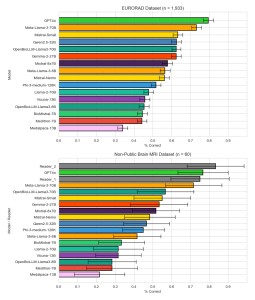
A moderate positive correlation between model size and accuracy was determined (Pearson correlation coefficient r = 0.54). LLMs fine-tuned with domain-specific training data showed lower accuracy compared to general-purpose models of comparable size.
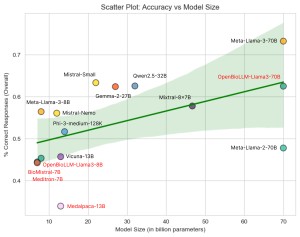