Results:
Significant inter-reader variability among human readers with poor to moderate agreement (κ=-0.018 to κ=0.41) was observed, with some readers showing more homogenous interpretations of quality features and overall quality than others. Interestingly, we did not find evident direct correlations between individual experience or background and the differences in ratings. In comparison, the AI software demonstrated higher consistency with fewer outliers (positive as well as negative), highlighting its generalization capability.
For a comprehensive evaluation of overall image quality, we conducted an analysis of agreement across all readers, as illustrated in Figure 1. The highest recorded accuracy, 61%, was observed between reader 1 and reader 2, while the lowest, 26%, occurred between reader 1 and reader 5, as well as between the b-box software and reader 5.
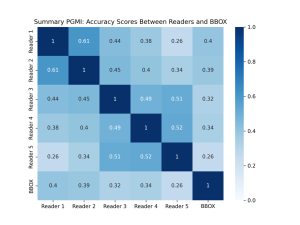
Figure 2 provides a visual representation of the disparities in Cohen’s kappa resulting from subtracting the mean human inter-reader agreement from the mean software performance (compared to human readers). It elucidates instances where the software outperforms or falls behind human performance. It surpassed human inter-reader agreement in detecting medial glandular tissue cuts (mean Cohen’s kappa: 0.43 (software) vs. 0.36 (experts)), mammilla deviation (mean Cohen’s kappa: 0.26 (software) vs. 0.24 (experts)), pectoral muscle detection (mean Cohen’s kappa: 0.72 (software) vs. 0.70 (experts)), and pectoral angle measurement (mean Cohen’s kappa: 0.49 (software) vs. 0.42 (experts)). For the remaining features, the software exhibited performance comparable to human assessment, with the highest difference measured at 0.18 for the PNL PGMI feature.
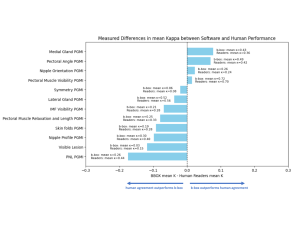