Retrospective, multicentric brain MRI data from three different phase II and III multicenter clinical trials in neuro-oncology [7-10], alongside retrospective institutional data (n=2179 patients with glioblastoma, n=8544 examinations, n=63327 sequences) from 249 hospitals and 29 scanner types, with magnetic field strengths ranging from 1 to 3 T, were used to develop a network based on ResNet-18 architecture. The following target MRI sequences were selected: T1, postcontrast T1 (CT1), T2, fluid-attenuated inversion recovery (FLAIR), susceptibility-weighted imaging (SWI), apparent diffusion coefficient (ADC), diffusion-weighted imaging (DWI) sequences with low b-values (Low-B-DWI) and DWI sequences with high b-values (High-B-DWI). Furthermore, a “T2*/DSC-related” class was defined that included gradient recalled echo T2*-weighted imaging (T2*) and dynamic susceptibility contrast (DSC)-related sequence types.
The two-dimensional-midsection images from each sequence were allocated to training/validation (~80%) and testing (~20%) using a stratified split to ensure balanced groups across institutions, patients and MRI sequence types. The prediction accuracy was quantified for each sequence type and subgroup comparison of model performance was performed using χ2 tests.
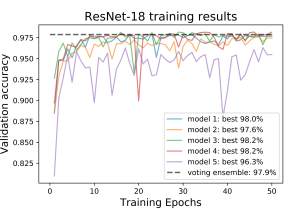